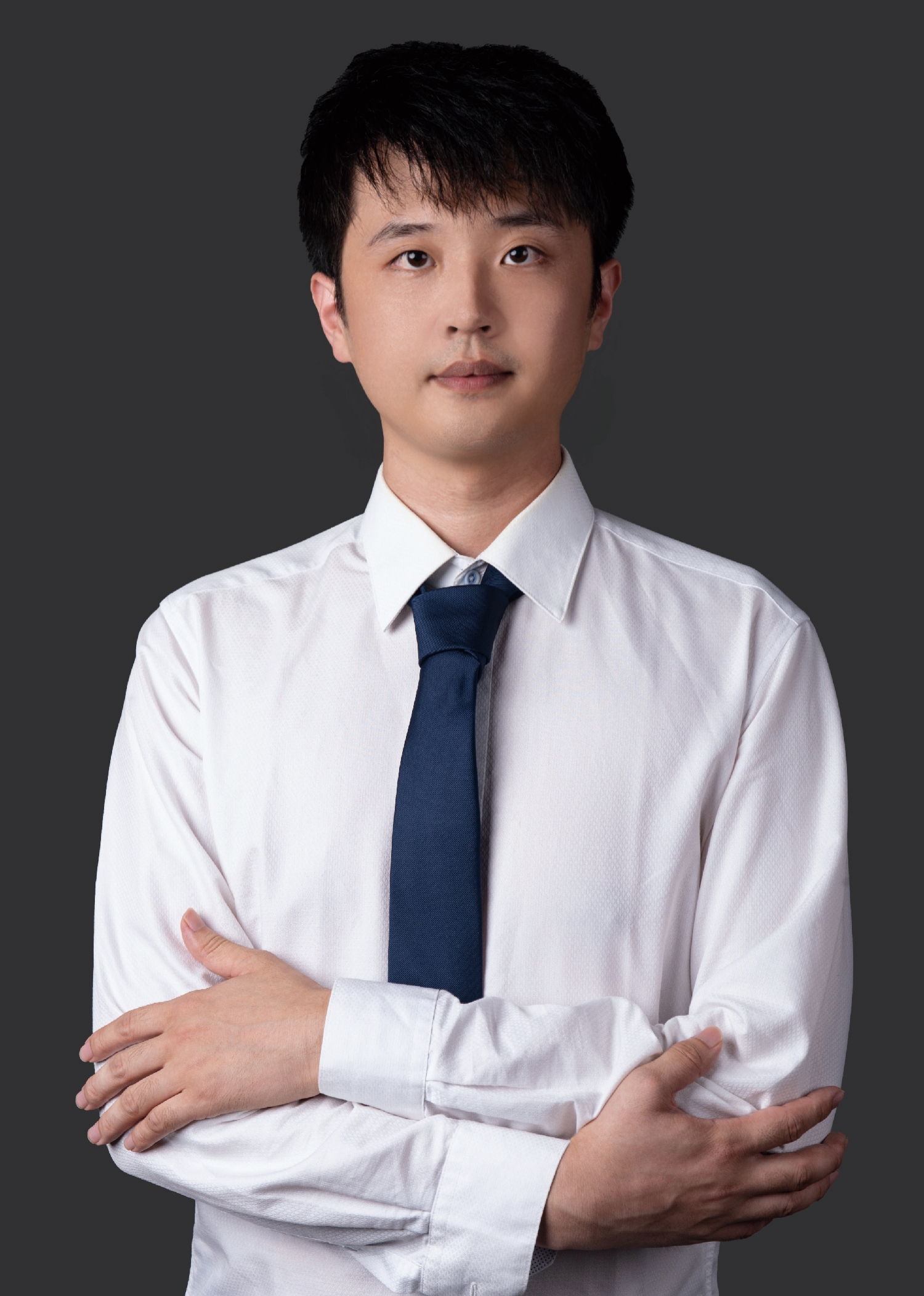
惠宇,男,1994年生,特聘副教授,硕士生导师。主要研究方向:自适应控制,数据驱动控制,学习控制。
2021年博士毕业于青岛科技大学,2021-2023年在北京航空航天大学从事博士后研究工作。
目前已发表高水平学术论文20余篇,其中SCI收录论文12篇、IEEE汇刊11篇。出版英文专著1部。申请发明专利6项,授权3项。担任International Journal of Machine Learning and Cybernetics、International Journal of Systems Science、Journal of Cleaner Production等国际期刊的审稿人。
主持国家自然科学基金青年基金项目1项,博士后科学基金面上项目1项,参与国家自然科学基金面上项目2项。获得获山东省研究生优秀成果奖一等奖,山东省优秀博士论文等荣誉。
招生方向:0811控制科学与工程、085406控制工程,欢迎同学们报考!
联系邮箱:yuhuisx@163.com。
代表性论著:
Yu Hui, Ronghu Chi, Biao Huang, and Zhongsheng Hou. Extended state observer-based data-driven iterative learning control for permanent magnet linear motor with initial shifts and disturbances, IEEE Transactions on Systems, Man, and Cybernetics: Systems, vol. 51, no. 3, pp. 1881-1891, 2021.
Yu Hui, Ronghu Chi, Biao Huang, Zhongsheng Hou, and Shangtai Jin. Observer-based sampled-data model-free adaptive control for continuous time nonlinear nonaffine systems with input rate constraints, IEEE Transactions on Systems, Man, and Cybernetics: Systems, vol. 51, no.2, pp. 7813-7822, 2021.
Yu Hui, Ronghu Chi, Biao Huang, and Zhongsheng Hou. 3-D learning-enhanced adaptive ILC for iteration-varying formation tasks, IEEE Transactions on Neural Networks and Learning Systems, vol. 31, no. 1, pp. 89-99, 2020.
Yu Hui, Ronghu Chi, Biao Huang, and Zhongsheng Hou. Data-driven adaptive iterative learning bipartite consensus for heterogeneous nonlinear cooperation-antagonism networks, IEEE Transactions on Neural Networks and Learning Systems, vol. 34, no. 11, pp. 8262 - 8270, 2022.
Yu Hui, Deyuan Meng, Ronghu Chi, and Kaiquan Cai. Sampled-data adaptive iterative learning control for uncertain nonlinear systems, IEEE Transactions on Systems, Man, and Cybernetics: Systems, to be published, Doi: 10.1109/TSMC.2024.3373588, 2024.
Yu Hui, Ronghu Chi, and Yang Liu. Data-based analysis of iterative learning control for MIMO nonaffine nonlinear systems with multiple nonrepetitive uncertainties, International Journal of Robust and Nonlinear Control, vol. 33, no. 12, pp. 6916-6931, 2023.
惠宇,池荣虎.基于迭代扩张状态观测器的数据驱动最优迭代学习控制.控制理论与应用,2018,35(11):1672-1679.